EC-DIT: Scaling Diffusion Transformers with Adaptive Expert-Choice Routing
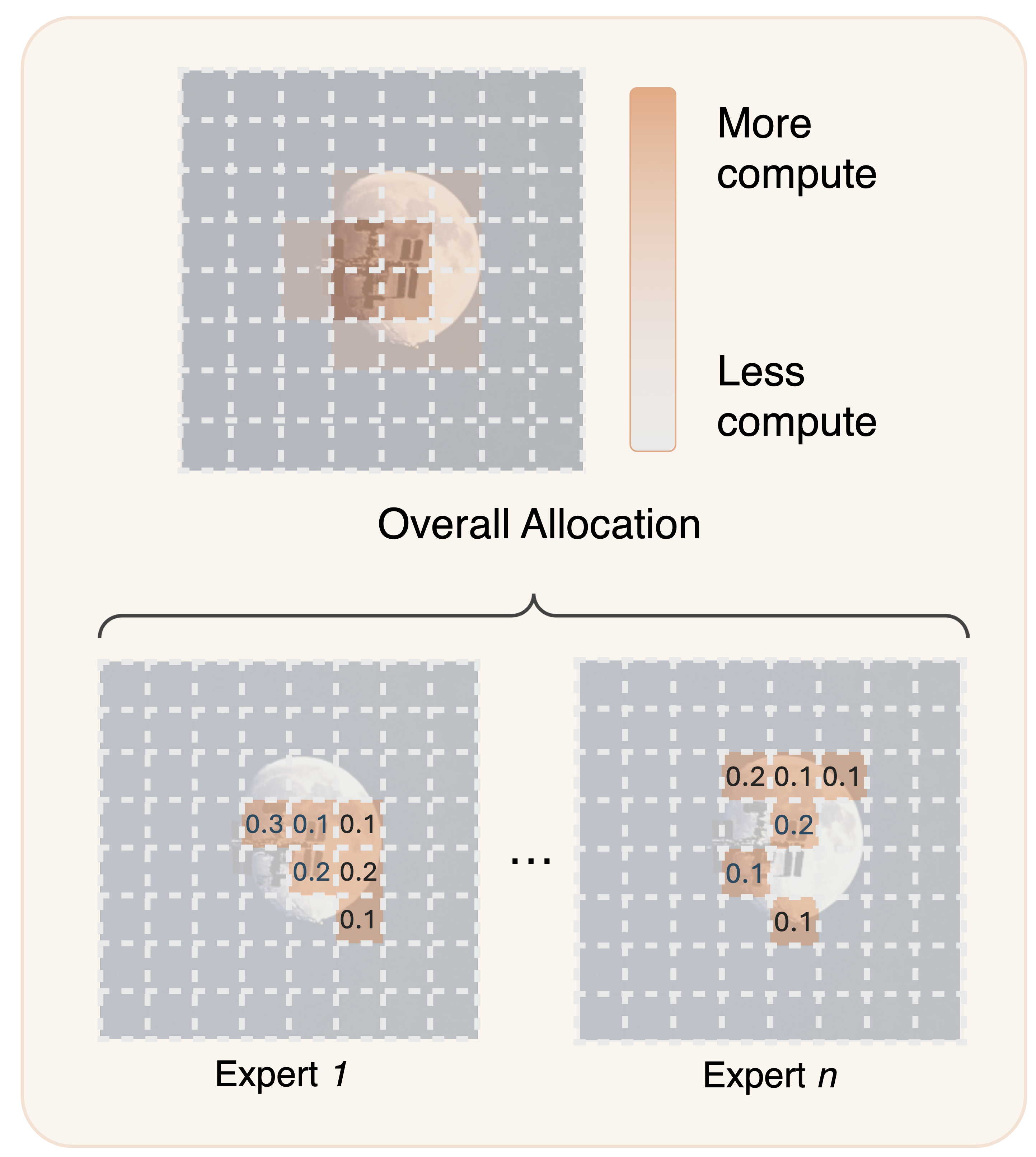
Abstract
Diffusion transformers have been widely adopted for text-to-image synthesis. While scaling these models up to billions of parameters shows promise, the effectiveness of scaling beyond current sizes remains underexplored and challenging. By explicitly exploiting the computational heterogeneity of image generations, we develop a new family of Mixture-of-Experts (MoE) models (EC-DIT) for diffusion transformers with expert-choice routing. EC-DIT learns to adaptively optimize the compute allocated to understand the input texts and generate the respective image patches, enabling heterogeneous computation aligned with varying text-image complexities. This heterogeneity provides an efficient way of scaling EC-DIT up to 97 billion parameters and achieving significant improvements in training convergence, text-to-image alignment, and overall generation quality over dense models and conventional MoE models. Through extensive ablations, we show that EC-DIT demonstrates superior scalability and adaptive compute allocation by recognizing varying textual importance through end-to-end training. Notably, in text-to-image alignment evaluation, our largest models achieve a state-of-the-art GenEval score of 71.68% and still maintain competitive inference speed with intuitive interpretability.
Materials
BibTeX
@misc{sun2024ecditscalingdiffusiontransformers,
title={EC-DIT: Scaling Diffusion Transformers with Adaptive Expert-Choice Routing},
author={Haotian Sun and Tao Lei and Bowen Zhang and Yanghao Li and Haoshuo Huang and Ruoming Pang and Bo Dai and Nan Du},
year={2024},
eprint={2410.02098},
archivePrefix={arXiv},
primaryClass={cs.CV},
url={https://arxiv.org/abs/2410.02098}
}