Two Birds with One Stone: Enhancing Calibration and Interpretability with Graph Functional Neural Process
Accepted at AISTATS'24,
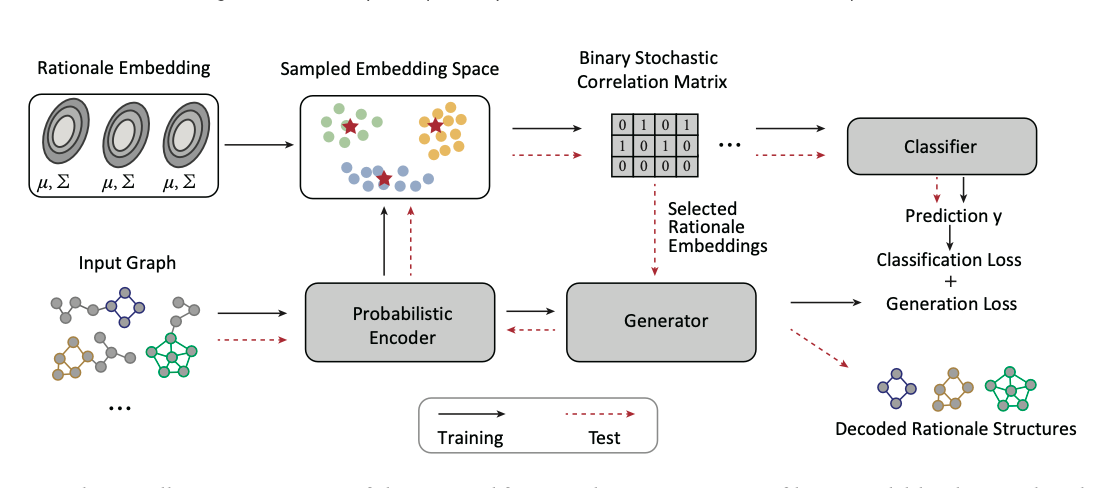
Abstract
- Proposed a generalized uncertainty-aware and interpretable graph classification model that combines graph functional neural process (FNP) and graph generative models to enhance calibration and interpretability;
- Improved the expected calibration error by up to 4.8% and rationale F1 score by up to 10.8% compared with the strongest baselines.
Materials
BibTeX
@article{pmlr-v238-kong24a,
title = { Two Birds with One Stone: Enhancing Uncertainty Quantification and Interpretability with Graph Functional Neural Process },
author = {Kong, Lingkai and Sun, Haotian and Zhuang, Yuchen and Wang, Haorui and Mu, Wenhao and Zhang, Chao},
booktitle = {Proceedings of The 27th International Conference on Artificial Intelligence and Statistics},
pages = {4582--4590},
year = {2024},
editor = {Dasgupta, Sanjoy and Mandt, Stephan and Li, Yingzhen},
volume = {238},
series = {Proceedings of Machine Learning Research},
month = {02--04 May},
publisher = {PMLR},
pdf = {https://proceedings.mlr.press/v238/kong24a/kong24a.pdf},
url = {https://proceedings.mlr.press/v238/kong24a.html},
}